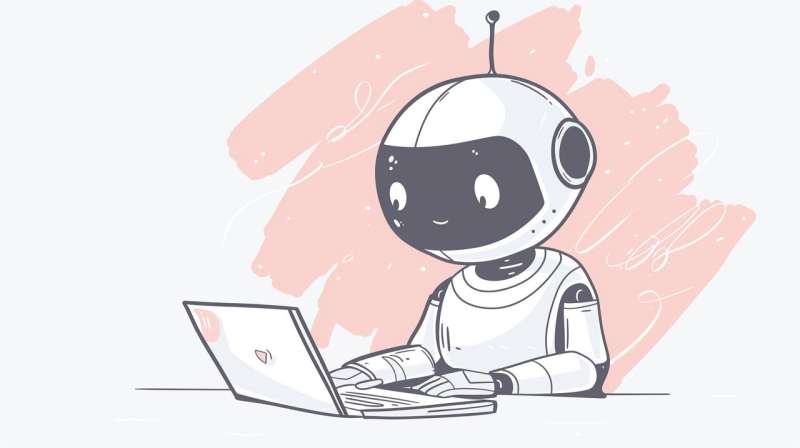
Credit: Public Pixabay / CC0 domain
During the summary of scientific studies, large-language models (LLM) as Chatgpt and Deepseek produce inaccurate conclusions in up to 73% of cases, according to a study by Uwe Peters (University of Utrecht) and Benjamin Chin-Yee (Western University, Canada / University of Cambridge, United Kingdom). The researchers tested the most important LLMs and analyzed thousands of scientific summaries generated by the chatbot, revealing that most models regularly produced wider conclusions than those of summary texts.
Surprisingly, invite precision Increased the more recent problem and LLM have made it possible to perform lower than the oldest.
The work is published in the newspaper Royal Society of Open Sciences.
Nearly 5,000 summaries generated by LLM analyzed
The study has evaluated what extent of ten leading llms, including Chatgpt, Deepseek, Claude and Llama, summarize the summaries and complete articles of Top Science and medical journals (For example, Nature,, ScienceAnd Lancet). Testing the LLM over a year, the researchers collected 4,900 summaries generated by LLM.
Six of the ten models systematically exaggerated the assertions found in the original texts, often in a subtle but impactful manner; For example, the change of prudent and past complaint as “the treatment was effective in this study” to a more swecing version and in the present as “the treatment is effective”. These changes can mislead readers by making believe that the results apply much more widely than they really do.
The precision invites us back
Surprisingly, when the models were explicitly caused to avoid inaccuracies, they were almost twice as likely to produce too genual conclusions when they received a simple summary request.
“This effect is worrying,” said Peters. “Students, researchers and decision -makers can assume that if they ask Chatgpt to avoid inaccuracies, they will get a more reliable summary. Our results prove the opposite.”
Do humans do better?
Peters and Chin-Yee also directly compared the summaries generated by Chatbot with summaries of the same articles. Unexpectedly, chatbots were almost five times more likely to produce great generalizations than their human counterparts.
“Worry,” said Peters, “new AI models, like Chatgpt-4o and Deepseek, have obtained worse than older results.”
Reduce
Researchers recommend using LLM such as Claude, which had the greatest generalization precision, defining chatbots to reduce “temperature” (the parameter fixing the “creativity” of a chatbot) and using invites that apply indirect reports and in the past in scientific summaries.
Finally, “if we want AI to support scientific literacy rather than undermining it,” said Peters, “we need more vigilance and testing of these systems in scientific communication contexts.”
More information:
Uwe Peters et al, generalization bias in the model of large language of scientific research, Royal Society of Open Sciences (2025). DOI: 10.1098 / RSOS.241776
Supplied by
Utrecht University
Quote: Important chatbots regularly exaggerated scientific results, shows the study (2025, May 13) recovered on May 14, 2025 from https://phys.org/News/2025-05-Prominent-Chatbots-Routinery-Exagéate-science.html
This document is subject to copyright. In addition to any fair program for private or research purposes, no part can be reproduced without written authorization. The content is provided only for information purposes.