The use of artificial intelligence (AI) explodes through many branches of science. Between 2012 and 2022, the average proportion of scientific articles engaging with AI, in 20 areas, quadrupled (see “Rise of AI research”), including economics, geology, political science and psychology1.
Hopes are high that AI can accelerate scientific discovery, because the rate at which fundamental progress is made seems to slow down: Although there is more funding, publications and staff, we make progress at a slower rate.
But the haste to adopt AI has consequences. As its use proliferates – in the forecasting of disease epidemics, forecasting the results of people’s lives and the anticipation of civil wars – a certain degree of prudence and introspection is justified. While statistical methods in general include a risk of being used wrongly, AI has even greater risks due to its complexity and black nature. And errors are becoming more and more common, especially when standard tools are used by researchers who have limited computer expertise. It is easy for researchers to overestimate the predictive capacities of an AI model, thus creating the illusion of progress while blocking real progress.
Here we discuss dangers and suggest a set of remedies. Establish clear scientific guidelines on how to use these tools and techniques is urgent.
There are many ways that can be deployed in science. It can be used to effectively comb previous work or to search for a problem space (for example, drug candidates) for a solution which can then be verified by conventional means.
Another use of AI is to build an automatic learning model for a phenomenon of interest and question it to acquire knowledge of the world. Researchers call this science based on automatic learning; It can be considered as an upgrade of conventional statistical modeling. Automatic learning modeling is the ax chainsaw by hand of statistics – powerful and more automated, but dangerous if it is poorly used.
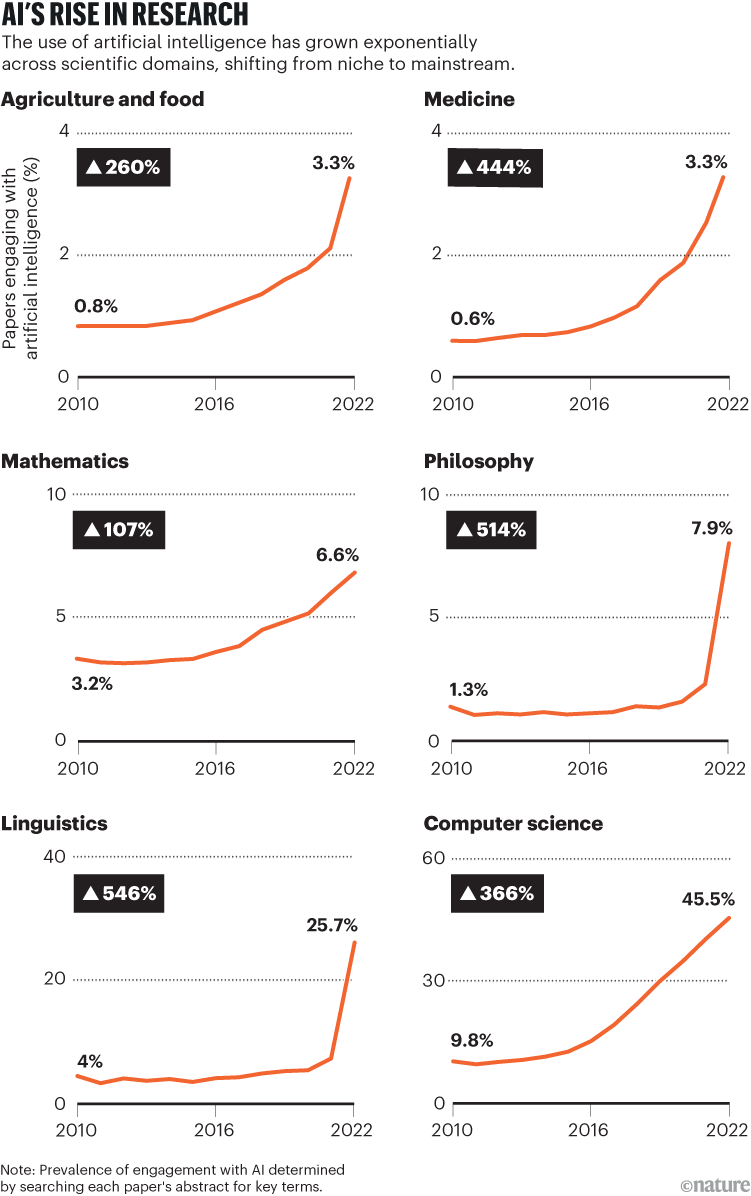
Source: Ref. 1
Our concern mainly concerns these approaches based on the model, in which AI is used to make predictions or test the hypotheses on the operation of a system. A common source of error is “leak”, a problem that arises when information from the evaluation data of a model does not influence its training process. When this happens, an automatic learning model can simply memorize models in evaluation data instead of capturing significant models behind the phenomenon of interest. This limits the applicability of the real world of these models and produces little scientific knowledge.
Thanks to our research and the compilation of existing evidence, we found that articles in at least 30 scientific areas – ranging from psychiatry and molecular biology to IT security – which use automatic learning are prey to leaks2 (see Go.nature.com/4ieawbk). It is a type of “test teaching”, or worse, to give the answers before the exam.
For example, during the COVID-19 pandemic, hundreds of studies said that AI could diagnose the disease using chest radiographs or computed tomography. A systematic review of 415 of these studies revealed that only 62 met the basic quality standards3. Even among them, the faults were widespread, in particular bad evaluation methods, double data and a lack of clarity on the question of whether “positive” cases came from people with confirmed medical diagnosis.
In more than a dozen studies, the researchers had used a set of training data in which all convinced cases were in adults, and the negatives were in children aged one to five. Consequently, the AI model had simply learned to distinguish adults and children, but the researchers wrongly concluded that they had developed a COVVI-19 detector.
It is difficult to systematically catch errors like these, because the evaluation of predictive precision is notoriously delicate and, for the moment, lack of normalization. IT code bases can last thousands of lines. Errors can be difficult to spot and only one error can be expensive. Thus, we think that the crisis of reproducibility in science based on machine learning is still in its infancy.
With some studies that now use major language models in research – for example, using them as substitutes for human participants in psychological experiences – there are even more ways whose research could be irreproductible. These models are sensitive to entries; Small changes in the invitation wording can cause notable changes to outputs. And because the models are often held and exploited by private companies, access can be limited at any time, which makes these studies difficult to reproduce.
We deceive
A greater risk of the hasty adoption of AI and automatic learning lies in the fact that the torrent of results, even without error, may not lead to real scientific progress.
To understand this risk, consider the impact of an extremely influential article in 2001, in which the statistician Leo Breiman cleverly described the cultural and methodological differences between the fields of statistics and automatic learning4.
Does AI compromise scientific photography? There is still time to create an ethical code of conduct
He firmly recommended the latter, in particular the adoption of automatic learning models on simpler statistical models, with priority predictive precision on the questions of the reliability of the model represents nature. In our opinion, this plea did not mention the known limits of the automatic learning approach. Paper does not make enough distinction between the use of automatic learning models in engineering and in natural sciences. Although Breiman has noted that these black box models can work well in engineering, as by classifying submarines using Sonar data, they are more difficult to use in natural sciences, in which nature explains (say, principles behind the propagation of sound waves in water) is the interest.
This confusion is still widespread and too many researchers are seduced by the commercial success of the AI. But just because an approach to modeling is good for engineering, that does not mean that it is good for science.
There is an old maxim that “each model is false, but some models are useful”. It takes a lot of work to translate the results of the models to the affirmations of the world. The automatic learning toolbox facilitates the creation of models, but it does not facilitate the end of knowledge of the world and could well make it more difficult. Consequently, we run the risk of producing more but understanding less5.
Science is not just a collection of facts or discoveries. Real scientific progress occurs through theories, which explain a collection of results and paradigms, which are conceptual tools to understand and study an area. While we go from results to theories, including paradigms, things become more abstract, wider and less accelerated for automation. We suspect that the rapid proliferation of AI -based scientific results has not accelerated – and could even have inhibited – these higher levels of progress.
If researchers in a field are concerned with defects in individual articles, we can measure their prevalence by analyzing a sample of articles. But it is difficult to find evidence of smokers that the scientific communities as a whole are too important to predictive precision to the detriment of understanding, because it is not possible to access the counterfactual world. That said, historically, there have been many examples of fields stuck in a rut even if they exceeded in the production of individual results. Among them, alchemy before chemistry, astronomy before the copernican revolution and geology before plates tectonics.
A cry for AI studies that do not make headlines
The history of astronomy is particularly relevant for AI. The model of the universe with the earth in its center was extremely precise to predict planetary movements, due to stuff such as “epicycles” – the hypothesis that the planets move in circles whose centers revolve around the earth along a wider circular path. In fact, many modern planetarium projectors use this method to calculate trajectories.
Today, AI excels in producing the equivalent of epicycles. Everything else being equal, being able to tighten more predictive juice from erroneous theories and inadequate paradigms will help them to stay longer, hindering real scientific progress.
The paths forward
We have highlighted two main problems with the use of AI in science: defects in individual studies and epistemological problems with the large adoption of AI.
Here are provisional ideas to improve the credibility of scientific studies based on automatic learning and avoid illusions of progress. We offer them as a starting point for the discussion, rather than proven solutions.